Deep learning algorithms have gained importance in astroparticle physics in the last years. They have been shown to outperform traditional strategies in particle identification, tracking and energy reconstruction.The attractive feature of these techniques is their ability to model large dimensionality inputs and catch non-trivial correlations among the variables, which could be hidden or not easy to model.
After the launch of CSES [1], a Deep Learning based event reconstruction has been developed for electrons and protons measured by Limadou HEPD [2]. The reconstruction chain is formed by a set of three deep neural networks which takes as input the signals produced by the detector. They separate between electrons and protons and reconstruct the arrival direction and the kinetic energy of incoming particles. A dedicated Monte Carlo simulation of electrons from [0,200] MeV and of protons from [0,1000] MeV was produced to train these networks.
They showed superior performances once compared to the standard methods and extend the reconstruction for particles not contained in the apparatus. For that reason this tool has been integrated in the official reconstruction software used by the collaboration
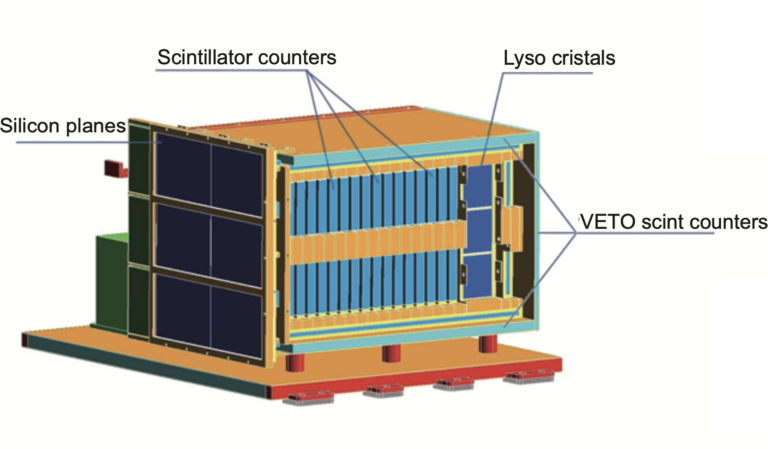
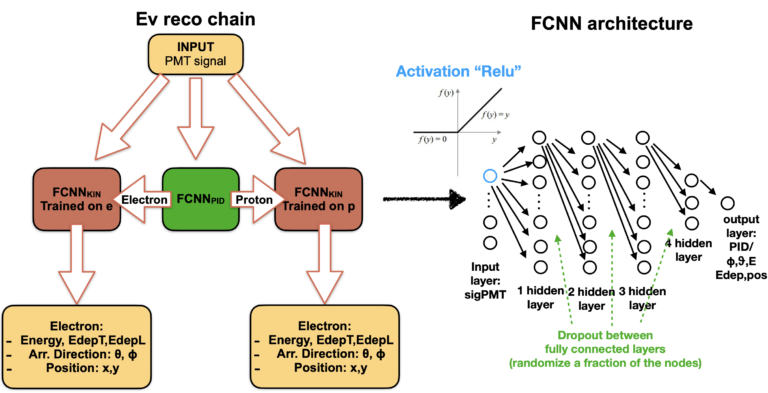
For the next generation of the experiment that will be launched on board of CSES-02 in 2022, an event reconstruction fully based on Deep Learning have been commissioned and preliminary studies are ongoing.
[1] CSES website, http://cses.roma2.infn.it/
[2] “The HEPD particle detector of the CSES satellite mission for investigating seismo-associated perturbations of the Van Allen belts”, The Limadou Collaboration, https://link.springer.com/article/10.1007/s11431-018-9234-9